Understanding Data Labeling: The Backbone of AI and Machine Learning
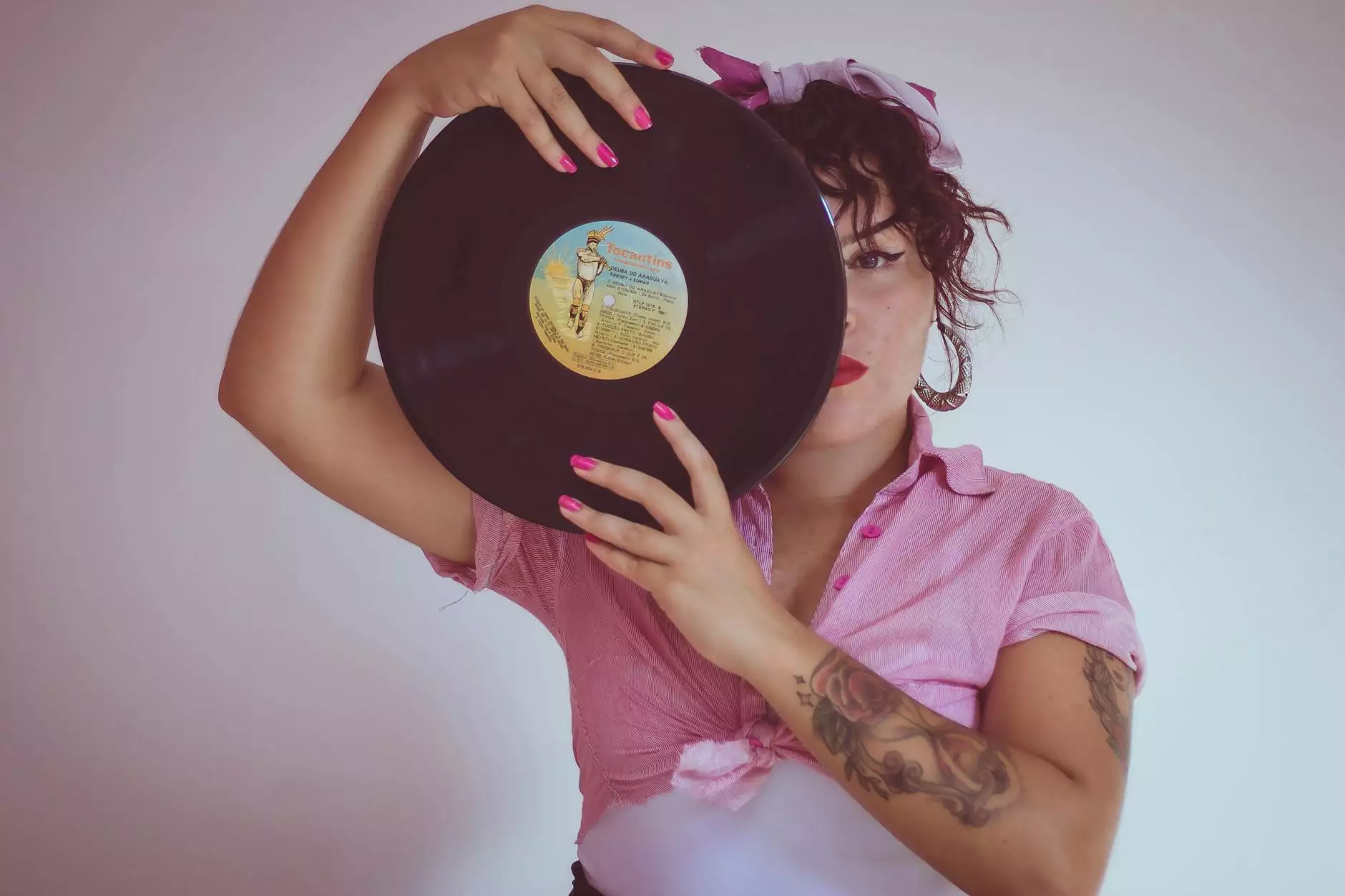
Data labeling has emerged as a critical component in the realm of artificial intelligence (AI) and machine learning (ML). As the amount of data generated each day continues to skyrocket, the demand for structured data that can train machine learning models also grows exponentially. This article delves deep into the concept of data labeling, its significance, and the platforms like KeyLabs.ai that provide essential tools and services for effective data annotation.
What is Data Labeling?
Data labeling refers to the process of identifying and tagging data with relevant labels to make it understandable to machine learning algorithms. This process is crucial, as supervised learning models rely on labeled datasets to learn patterns and make predictions. The labels can be simple categorizations, such as identifying objects in an image, or more complex annotations, such as sentiment analysis in text data.
The Importance of Data Labeling
The significance of data labeling cannot be overstated. It serves several key functions:
1. Training Machine Learning Models
Machine learning models are taught to recognize patterns within data through the training process. This training heavily relies on labeled data to provide clear examples of the output that corresponds to given inputs.
2. Enhancing Accuracy
High-quality labeled data directly impacts the accuracy of machine learning models. Well-labeled datasets enable algorithms to learn nuances and make better predictions, thus improving their overall performance.
3. Fostering Innovation and Development
In industries like healthcare, finance, and autonomous driving, advancements depend on the ability to train AI systems with vast amounts of accurately labeled data, driving innovations that improve efficiencies and outcomes.
Types of Data Labeling
There are various methods of data labeling, each suitable for different types of data and machine learning tasks:
- Image Annotation: Tagging images with relevant labels, including bounding boxes for object detection and segmentation masks for pixel-level classification.
- Text Annotation: Identifying sentiments, entities, and parts of speech in text to aid in natural language processing (NLP) applications.
- Video Annotation: Labeling frames in a video for tasks such as action recognition or object tracking.
- Audio Annotation: Transcribing and tagging audio clips for applications in speech recognition and audio classification.
The Data Labeling Process
The data labeling process can be broken down into several critical steps to ensure quality and efficiency:
1. Data Collection
Gather your data from various sources, ensuring it is relevant to the task at hand. This data can come from public datasets, proprietary collections, or generated data.
2. Preprocessing
Clean and prepare the data for labeling. This step may include removing duplicate entries, normalizing formats, or splitting data into manageable chunks.
3. Annotation
Utilize skilled annotators or automated tools to assign labels to the data. This process must be conducted with attention to detail to ensure data integrity.
4. Quality Assurance
Implement quality checks to review the labeled data for accuracy. This may involve cross-referencing with existing annotations or conducting peer reviews.
5. Feedback Loop
Establish a feedback mechanism where annotators can learn from corrections and improve future labeling tasks.
Challenges in Data Labeling
Despite its importance, data labeling comes with its fair share of challenges:
1. Scalability
As the volume of data grows, scaling the labeling process to accommodate vast datasets becomes increasingly complex.
2. Quality Control
Ensuring the accuracy and consistency of labels across large datasets can be a daunting task, often requiring extensive training for annotators.
3. Subjectivity
Some labeling tasks require subjective judgment, leading to variations in how different annotators might label the same data.
Automating Data Labeling with Tools and Platforms
To address the challenges associated with data labeling, many companies are turning to specialized platforms that offer annotation tools and services:
KeyLabs.ai: Leading the Charge
KeyLabs.ai stands out in the crowded field of data annotation platforms by offering cutting-edge tools for data labeling. Here’s how KeyLabs.ai differentiates itself:
- User-Friendly Interface: KeyLabs.ai fosters productivity with a straightforward and intuitive user interface, allowing annotators to focus on quality labeling.
- Automation Capabilities: Employs advanced machine learning algorithms to automate repetitive labeling tasks, expediting the process without compromising accuracy.
- Collaboration Features: Facilitates seamless collaboration among teams, providing dashboards that track progress and provide real-time feedback.
- Integration Flexibility: Easily integrates with various data sources and machine learning frameworks, making it a versatile choice for organizations.
The Future of Data Labeling
The future of data labeling holds exciting possibilities as automation and machine learning continue to evolve. We can expect:
1. Increased Efficiency
With ongoing advancements in AI, we can anticipate an increase in automated labeling solutions that will significantly enhance efficiency in the labeling process.
2. Enhanced Quality Control
AI-driven quality control mechanisms can improve the consistency and accuracy of labeled data, providing a more reliable foundation for training machine learning models.
3. Greater Accessibility
As platforms like KeyLabs.ai become more user-friendly and affordable, we can expect a broader range of organizations, from startups to large enterprises, to access high-quality data labeling tools.
Conclusion
In conclusion, data labeling is an indispensable element of the AI and machine learning landscape. As the demand for labeled data continues to escalate, understanding and optimizing the labeling process is crucial for organizations aiming to leverage AI technologies effectively. By utilizing platforms like KeyLabs.ai, businesses can streamline their annotation processes, improve data quality, and ultimately drive innovation in their respective fields. Investing in efficient data labeling is not just an operational move; it is a strategic necessity for companies looking to thrive in the data-driven age.